- Portfolio News
- 04 March, 2025
As investors, we’ve been actively exploring how AI is transforming entire industries and functions. Few areas hold as much promise for improving human wellbeing as drug R&D and healthcare. The pharmaceutical industry faces daunting statistics: a 90–95% failure rate, with development costs climbing and returns on investment shrinking.
However, we’ve long believed in the potential of AI applied to the biological domain — to help researchers make connections across large and heterogeneous data and therefore uncover new insights into complex biological mechanisms that we still only partly understand. Based on this thesis, we have previously backed companies like Healx, Sophia Genetics and Rahko.
Last month, we were excited to bring together founders, researchers, and operators from the biotech community and host a panel with two pioneers in the field:
- Max Jaderberg – Chief AI Officer at Isomorphic Labs
- Tim Guilliams – Founder & CEO at Healx
Below are some of our key takeaways from the discussion:
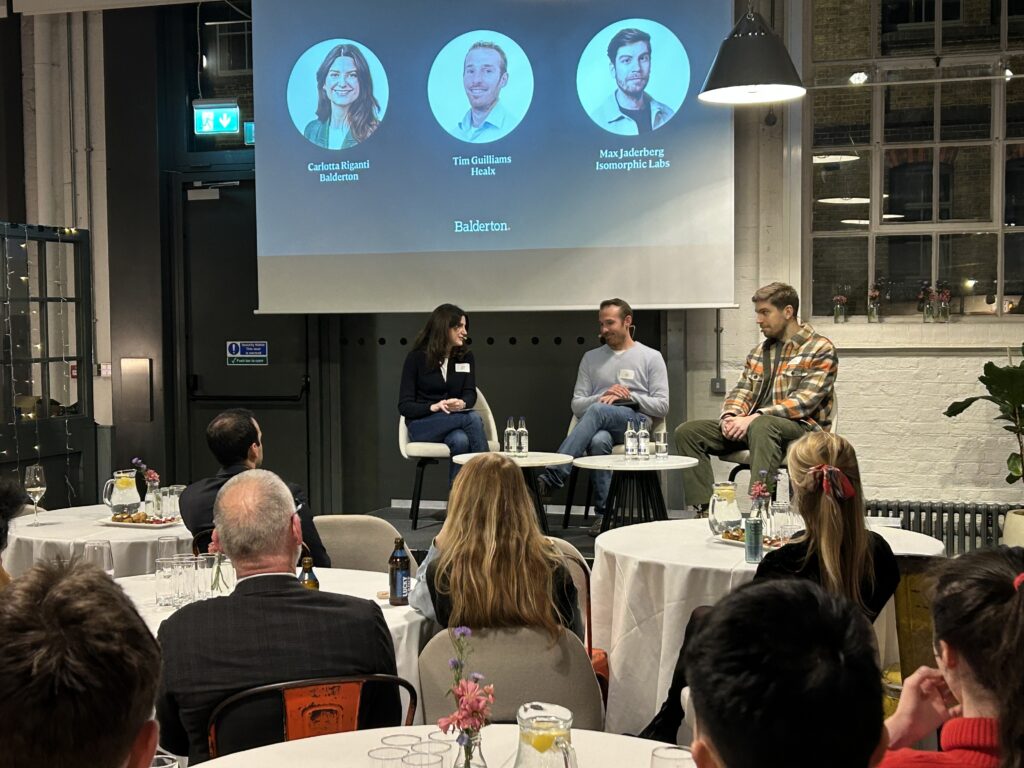
Reimagining the drug discovery pipeline.
Companies like Healx and Isomorphic are rethinking every step in the drug discovery process from first principle, and embedding computational methodologies and AI throughout. Their aim is to accelerate discovery by orders of magnitude, not just incrementally. For example, Healx advocates moving beyond the single-target paradigm, using AI to match targets and drugs in a parallelized way. They have advanced a candidate into Phase 2 clinical trials in the realm of rare diseases, where data is notoriously scarce. In the long term, Isomorphic envisions a world of open-ended, in-silico research, shifting away from lab research as much as possible. They’re starting pragmatically by focusing on the molecule design process, then progressively tackling additional bottlenecks.
Adapting AI architectures to biology and chemistry.
Unlike large language models trained on massive text corpora from the internet, biology and chemistry lack similarly scaled datasets. As a result, direct application of LLMs architecture has limitations. Instead, we’re seeing tailored architectures that attempt to embed domain-specific knowledge—like AlphaFold, which uses a 2D interaction grid for input sequence, compared to a flat transformer. Still, we need to remain honest about how generalisable the resulting models really can be.
A more level playing field for startups.
This field may be more accessible to startups than one might think. AlphaFold began at a hackathon and a good enough amount of data exists in the public domain—though it often requires a lot of cleaning and preparation. As next step, startups should think through their own data strategy, looking at partnerships and possibly their own generation of proprietary, ML-ready datasets, to enable differentiated insights. Data scaling laws are beginning to emerge, meaning companies can start assessing the ROI of acquiring or generating incremental data.
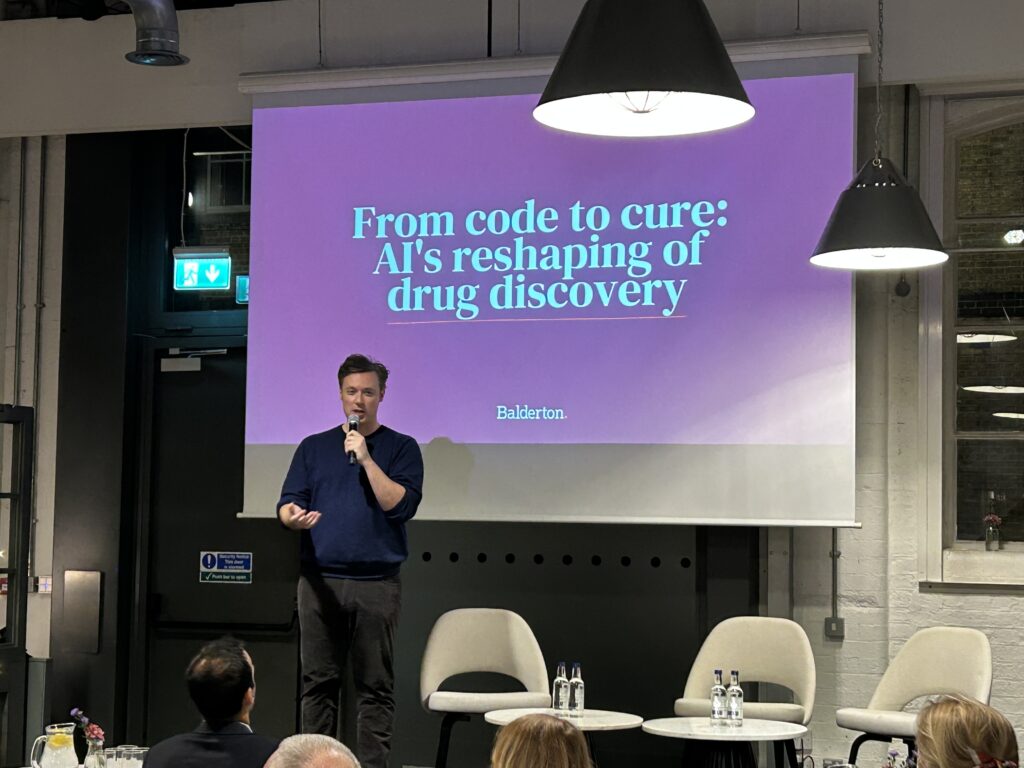
What’s the right business model?
The debate today continues: should AI-driven drug discovery companies behave more like software platforms or like biotech companies developing their own pipeline? Each model has pros and cons, and success stories are still limited on both sides. Currently, value is still anchored in IP rather than software (particularly outside of core infrastructure software and cloud). Yet we remain optimistic; technology shifts often lead to business model shifts, especially once initial successes emerge. Perhaps in time, it might become more common for software like platforms to capture the downstream value of drugs they help develop.
Practical advice for biotech founders.
For those building pipeline-centric companies, lessons learned include keeping org structures simple and cross-functional rather than department heavy, ensuring the team remains focused on advancing candidates. Innovate aggressively until you reach pre-clinical, but be as conservative as possible once you’re in clinical phases.
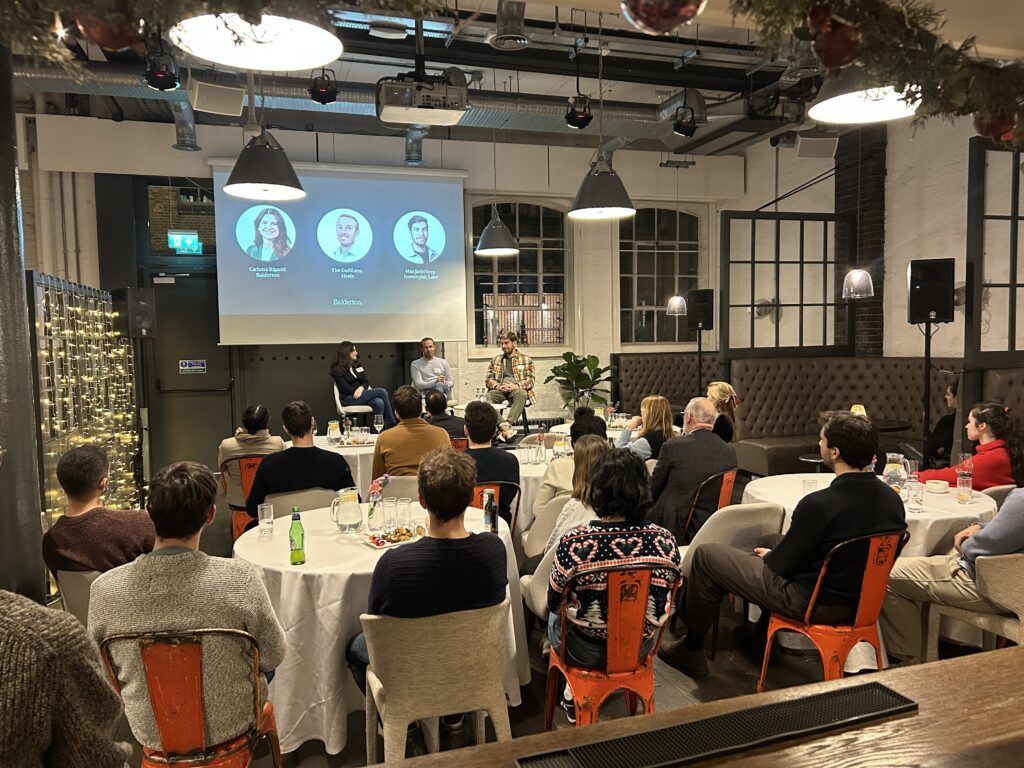
We’re excited to continue exploring how this field evolves. If you are innovating in the space, we’d love to say hello!